AI is revolutionizing industries at lightning speed, and drug discovery is emerging as one of its most game-changing applications. AI isn't just making R&D more efficient: it's transforming the way we discover and develop new drugs, improving targeting accuracy and speeding up the whole process. This evolution is reshaping the pharmaceutical landscape as never before. For investors, AI-driven drug discovery offers a unique and exciting opportunity, where cutting-edge technology meets the evolving healthcare sector, creating a space for growth and innovation.
In this article, we'll delve into the strategic advantages of AI in drug discovery, examine how it's redefining the pharmaceutical landscape, and showcase the key trends investors should be tracking.
The Role of AI in Drug Discovery
Let’s start from the top. AI-driven drug discovery refers to the application of machine learning (ML), deep learning, and other AI techniques to identify new drug candidates, optimize drug designs, predict clinical trial outcomes, and analyze massive biological datasets. By using AI, pharmaceutical companies are developing drugs faster and at lower costs compared to traditional R&D methods. A number of key areas have undergone drastic changes:
Target Identification – AI models sift through genomic data to identify potential biological targets for drug interventions, reducing the time needed to understand disease pathways.
Drug Design – AI-powered tools simulate molecular interactions, generating new drug candidates that exhibit high efficacy and low toxicity.
Preclinical Testing – AI helps in predicting the success of a drug in early testing phases, significantly reducing the likelihood of failure in costly later stages.
Clinical Trials – AI can optimize patient selection for trials, predict trial outcomes, and analyze real-time patient data, which increases the likelihood of approval.
Historically, drug discovery has been a time-consuming and costly process, with it taking an average of 10-15 years and over $2 billion to bring a new drug to market. But AI is turning the tables - in recent years, AI has made substantial progress in processing massive biomedical datasets, such as genomics and patient records, which allows researchers to uncover patterns and insights at an unprecedented scale. With AI platforms and algorithms continuously improving, it is expected that the future of drug discovery will see further efficiencies and breakthroughs.
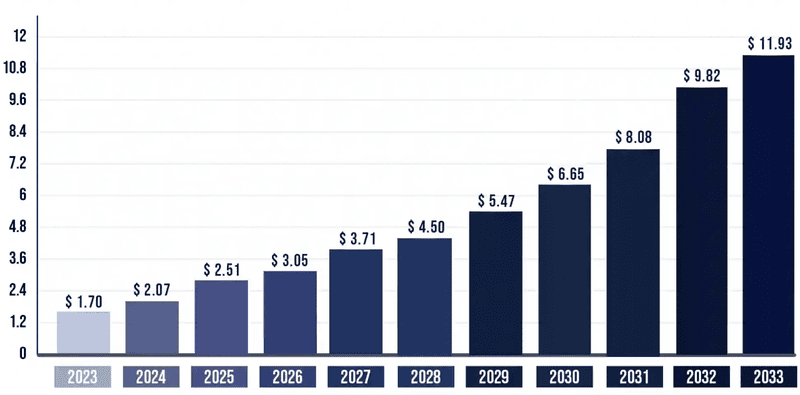
The market is showing that clearly: as of 2023, the global AI in drug discovery market was valued at approximately $1.7 billion,and is expected to grow at a CAGR of ca. 29.7% from 2024 to 2030. This exponential growth is boosted by the increasing need to accelerate drug development timelines, reduce costs, and enhance the precision of treatments. Investors are paying close attention to this space, recognizing the potential for AI to reshape the entire drug development pipeline, reduce the high failure rates in drug trials, and unlock new revenue streams through personalized medicine and rare disease treatments.
Global Demand Drivers
Rising Drug Development Costs - The traditional drug discovery process is extremely costly and time-consuming; introducing a new medicine typically costs over $1 billion and can take up to 14 years. AI has the potential to significantly reduce these costs and timelines.
Growing Adoption of AI Technologies - As of 2024, 95% of pharmaceutical companies are using AI in discovery phases. AI has saved drug developers 40% in costs through optimization in the discovery stages.
Increasing Investment and Funding - Between 2013 and 2018, over $7.20 billion was invested in AI within the pharmaceutical industry, across more than 300 deals.
Demand for Personalized Medicine - AI facilitates the development of personalized therapies by considering individual patient characteristics and genetic profiles.
Advancements in AI Technology - Continuous innovation in AI algorithms and computing power enhances the capabilities and accuracy of generative models.
High Attrition Rates in Drug Trials - Historically, clinical trials have only had a 10% success rate. AI is being leveraged to improve outcomes through better patient selection and monitoring.
Strategic Insights for Investors
Investment Opportunities in AI-Driven Drug Discovery
Drug Discovery and Design - Core AI technologies directly involved in identifying and designing new drug candidates, such as target identification and validation, small molecule design and optimization, biologics discovery (including antibodies, proteins, and peptides), or drug repurposing.
Predictive Analytics and Modeling - AI technologies that predict various aspects of drug behavior and efficacy, such as predictive toxicology and safety assessment, ADME (Absorption, Distribution, Metabolism, Excretion), or biomarker discovery and validation.
Clinical Trial Optimization - AI applications that improve the efficiency and success rate of clinical trials, such as clinical trial design and patient selection, real-world evidence analysis, or patient recruitment and retention.
Precision Medicine - AI technologies enabling personalized therapeutic approaches, such as pharmacogenomics, personalized drug dosing, or companion diagnostics development.
Data Integration and Analysis - AI tools that handle and interpret large, complex datasets, such as multi-omics data integration, Electronic Health Records (EHR) analysis, or Natural Language Processing (NLP) for scientific literature.
AI Infrastructure and Platforms - Underlying technologies and platforms that enable AI-driven drug discovery, such as end-to-end AI drug discovery platforms and AI Software and Tools for Drug Discovery.
Leading AI technologies in drug discovery
Deep Learning - This subset of machine learning excels at processing complex, high-dimensional biological data, enabling the discovery of new drug compounds and predicting their interactions with biological systems.
Neural Networks - Neural networks mimic the human brain's ability to recognize patterns, allowing AI systems to identify potential drug candidates by analyzing vast datasets, including molecular structures and genetic data.
Generative AI Models - These models, such as generative adversarial networks (GANs) and variational autoencoders (VAEs), are used to create novel molecular structures and predict how they will interact with targets, accelerating drug design.
Key Private Players
Xaira Therapeutics - Xaira Therapeutics is a biotechnology company focused on AI-enhanced drug design for complex diseases. The company's platform combines computational biology with machine learning to optimize the discovery and development of novel therapies. Xaira has secured over $1 billion in funding, with backing from leading investors such as Foresite Capital and GV.
Insitro - Insitro is a biotechnology company that utilizes AI and machine learning to accelerate drug discovery and development. Its platform integrates large-scale data analysis, genomics, and disease modeling to improve therapeutic discovery processes. Insitro has raised over $743 million in funding, with top investors including Andreessen Horowitz, ARCH Venture Partners, and Third Rock Ventures.
Atomwise - Atomwise is a pioneering AI-powered drug discovery company that uses deep learning to predict molecular interactions and identify potential drug candidates. Atomwise’s technology streamlines the drug development process by rapidly screening vast chemical libraries. The company has raised $174 million in funding, with investors such as BVF Partners and Monsanto Growth Ventures.
Risk factors
Data Quality Issues - The effectiveness of AI in drug discovery heavily relies on the quality of the data used to train models. Inaccurate, incomplete, or biased datasets can lead to flawed AI models that generate unreliable predictions. Additionally, noise and inconsistencies in data can skew outcomes, leading researchers to dismiss promising compounds or advance ineffective ones, ultimately jeopardizing the entire drug discovery process.
Regulatory Challenges - Navigating the regulatory landscape poses significant challenges for AI-driven drug discovery. Different regulatory agencies, such as the FDA in the United States and EMA in Europe, may have varying requirements for the validation and approval of AI technologies. This lack of standardization can create confusion and slow down the approval process for AI-enhanced therapeutics.
Long Timelines for Drug Approval - The drug approval process is notoriously lengthy and multifaceted, often taking several years to complete. This inherent complexity can hinder the integration of AI innovations, as the time required for clinical trials and regulatory submissions may outpace the rapid advancements in AI technology.
The Bottom Line
While AI is on everyone's lips, we understand that it can be difficult for investors to identify clear opportunities. In our view, AI-driven drug discovery is one of the most exciting and promising sectors. Unlike other more saturated sectors, this field is just beginning to reveal its transformative power. With the potential to revolutionize the way we develop new medicines and tackle complex diseases, AI in drug discovery represents an opportunity for those looking to harness the impact of AI in one of the world's most important industries.
At Acquinox, we are committed to identifying revolutionary opportunities in these emerging fields. Thanks to our in-depth in-house research, strategy and network, we are able to provide our clients with access to the players we deem to be the most promising.
Published by Samuel Hieber